In this article
Linde: AI-based plant control
"This project is a great example of how collaboration accelerates value creation. We receive support for machine learning from appliedAI, technical process knowledge from various departments of Linde Engineering, and operational knowledge from Linde Gas. Each party brings their best expertise." - Dr. Dexin Luo, Head of Artificial Intelligence Solutions & Technologies at Linde.
Linde, the world leader in industrial gases, joined the appliedAI initiative as a partner in 2017 to accelerate the use of AI. Linde is currently working to implement AI solutions in all business areas. This will increase productivity and competitiveness across the entire value chain and master the journey to AI maturity. Linde and appliedAI have worked on various cooperation projects since Linde became a partner of appliedAI. Some of these projects related to the overall ecosystem. For example, the AI delegation traveled to China and Canada, and employees were trained in workshops. In addition, Linde also used appliedAI's technical expertise in projects where AI applications were implemented.
So how is Linde using AI to make its industrial plants more efficient? And how does appliedAI support the optimization of plant operations? The following case study provides an insight into the collaboration between Linde and appliedAI in the field of artificial intelligence for plant operations.
Background of the project
Since its first AI pilot project in 2016, Linde has been actively developing and implementing AI solutions throughout its entire value chain: from sales to supply chain to operations. Linde is also engaged in longer-term AI research topics, such as optimizing the efficiency of over a thousand production facilities around the globe.
Linde has already integrated a variety of AI projects into its processes, focusing on professionalizing its AI product strategy and product management. The company has a central AI team developing innovative AI solutions and offering AI training to the company. Digital teams are also embedded in business units with AI development capabilities. Linde uses AI to gain a competitive advantage through increased efficiency.
To improve its AI maturity level, Linde has identified the following measures, including training and enabling employees and optimizing plant operations.
The identified measures
AI training for employees
Linde's first challenge was to train experts in the domains and operational employees on the topic of artificial intelligence. Previously, there was a lack of experts who were knowledgeable in both AI technology and plant operations. Therefore, there was a need for domain experts who could pinpoint where AI could be used to improve plant efficiency. Raising awareness of AI activities within the company was important to build understanding for AI and accelerate its implementation.
Optimizing plant operations and automating control systems
Linde was already using advanced technologies for plant operations, with a large portion of its production plants remotely controlled by Remote Operating Centers (ROCs). A joint team of Linde domain experts and appliedAI engineers identified further opportunities for improving plant control to make them more efficient. In response to requests from the business community, Linde decided to use artificial intelligence to push the boundaries of optimized plants even further.
The plant control system consists of low-level and high-level controls. The task of the former is to ensure the correct settings for individual valves as well as actuators. This is managed by a Distributed Control System (DCS). The mechanisms of high-level control, on the other hand, have the task of controlling the plant as a whole. The controllers must be tuned regularly, and from time to time the plant load must be changed. This is a task that still had to be done manually.
Setting up new parts of the plant manually, as well as maintaining and adjusting the plant control algorithms on a regular basis, was particularly time-consuming. Although high-level control tasks still need to be performed manually by employees to ensure that equipment is operating in unison and optimally, these tasks could be handled by AI. It would work more efficiently and accurately.
Because the control algorithms in ROCs are not self-learning, they require a variety of data to predict the behavior of a plant. This data is also critical to apply a specific strategy to control the valves. This is the only way to ensure that process values are close to target values and achieve optimal results.
Although this process works well, it can be further improved to make it more efficient and sustainable. AI has the potential to provide an adaptive system that learns the plant's behavior and responds even better in dynamic situations.
AI plant control can predict real-world plant behavior and respond to it directly. This would allow Linde to save significant amounts of energy and would have a reduced workload. At the same time, better and more energy-efficient results would be achieved.
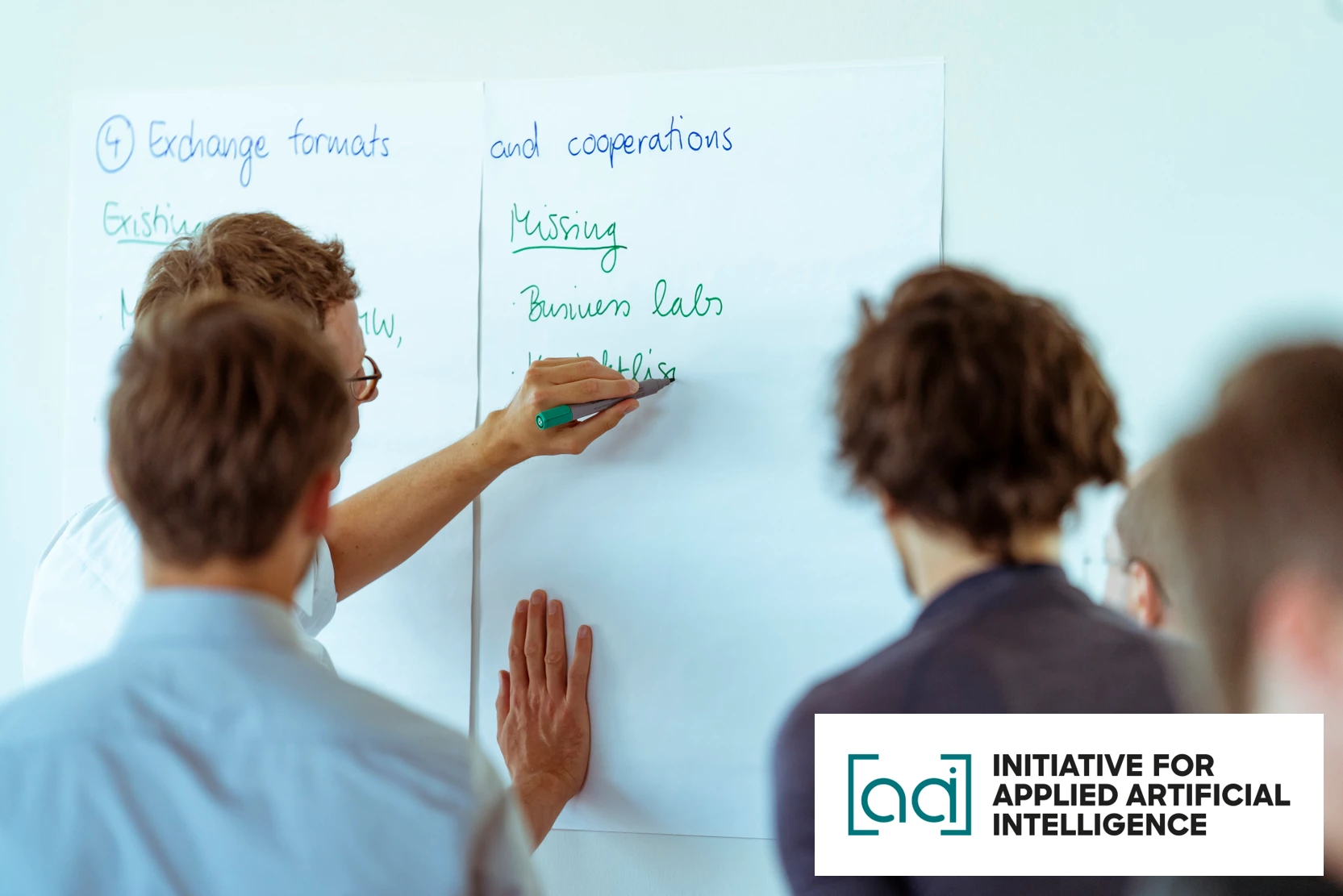
Approach and Methodology
After identifying the problems and areas in need of improvement, appliedAI and Linde began taking multiple important measures. One of the first actions taken by appliedAI was to train Linde employees in the field of AI through various workshops and trainings. Additionally, the team from appliedAI worked closely with Linde's highly experienced engineers and specialists to develop a reinforcement learning-based AI-controlled facility.
To sustain the momentum of these initiatives, appliedAI and Linde created a KI-based Lego KI Wonderland. This communicates the AI vision and raises awareness of AI activities across Linde's business areas in the public and among employees.
The following sections provide insight into the aforementioned collaborative projects supporting Linde in achieving its goals.
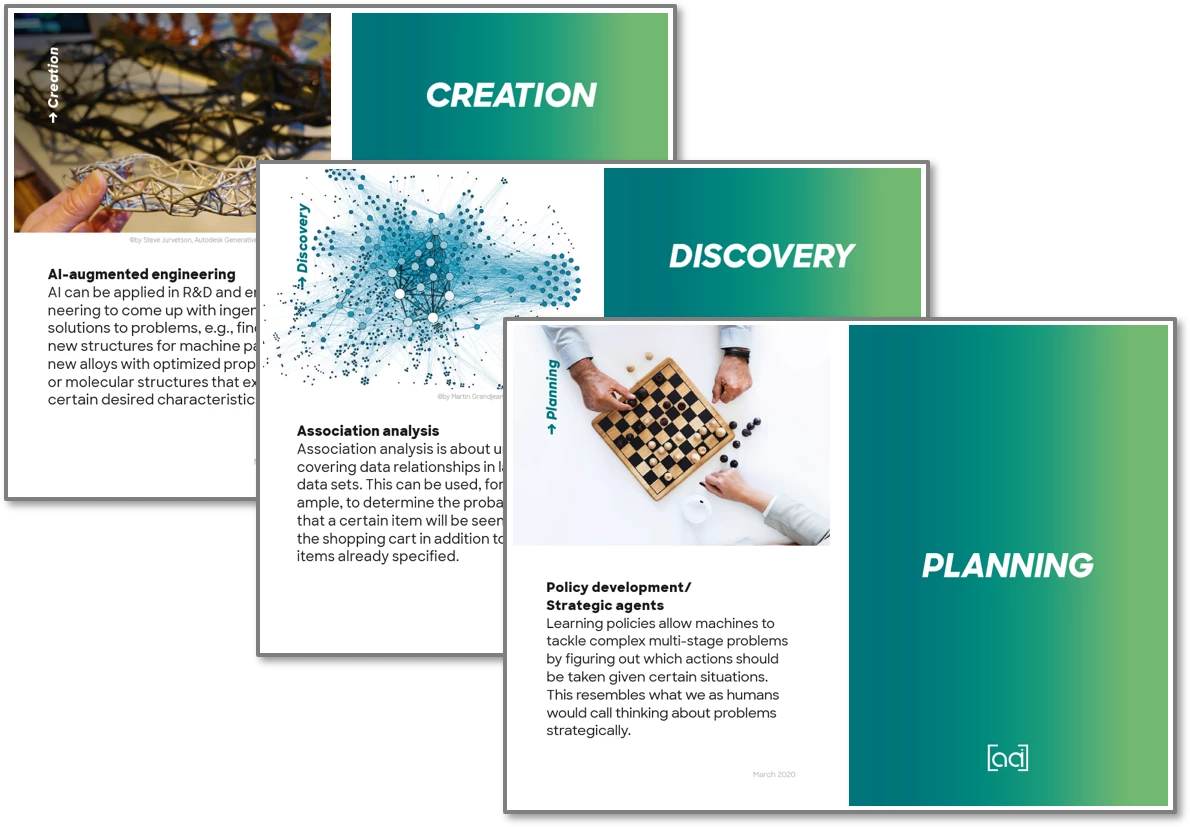
Workshops and AI tools for Linde
In order to enable the employees of Linde to identify and initiate AI use cases, they participated in several AI workshops and meetups by appliedAI. In general, the courses offered by appliedAI aim to support companies in further educating their employees and enable organizations to master the journey to AI maturity and implement AI faster. Linde employees participated in AI introduction courses, AI strategy courses, and project management workshops.
So far, Linde employees have participated in AI introduction courses held as part of the partnership with appliedAI. The AI introduction course provided an intensive and practical introduction to the field of Artificial Intelligence from various perspectives. The aim of this workshop was to create a shared understanding among employees of what AI is about. Additionally, they were equipped with basic knowledge about AI that they can use to determine where AI technology can be applied in their daily work.
At the workshop, employees looked at AI from a technical perspective. As a result, they became better equipped to have meaningful conversations about the use of AI at Linde. In addition, Linde employees participated in AI strategy courses that help companies develop their AI strategy. The strategy courses highlighted the opportunities and challenges of AI technologies.
Linde employees learned about the elements of comprehensive AI strategies, helping Linde to flesh out and further develop its AI vision. They were also equipped with relevant prerequisites for the successful use of AI in their company and learned how to assess companies' current AI maturity levels.
Participation in AI project management workshops helped employees gain a deeper understanding of how to manage AI projects. The workshops provided participants with a comprehensive overview of the complete AI project lifecycle and the most common obstacles encountered during each project phase. The workshops empowered employees to apply best practices and process models to manage AI projects. They learned how to:
- Identify profiles of key roles for different project phases,
- Avoid typical pitfalls when implementing AI projects,
- Identify different software and hardware requirements for different AI project types.
Linde also participated in appliedAI's AI delegation trip to China in 2018 and delegation trips to Canada and Singapore in 2019. Their goal was to build connections with AI companies and startups in the Canadian AI ecosystem and to represent the AI ecosystem from a German perspective.
Using the insights gained in the workshops and their internal expertise, employees were able to identify and create high-value AI use cases for Linde. In doing so, Linde used the AI use case playing cards developed by appliedAI's Strategy team. The cards are designed to help appliedAI partners conduct workshops and create AI use cases themselves. They helped Linde ideate its own AI use cases.
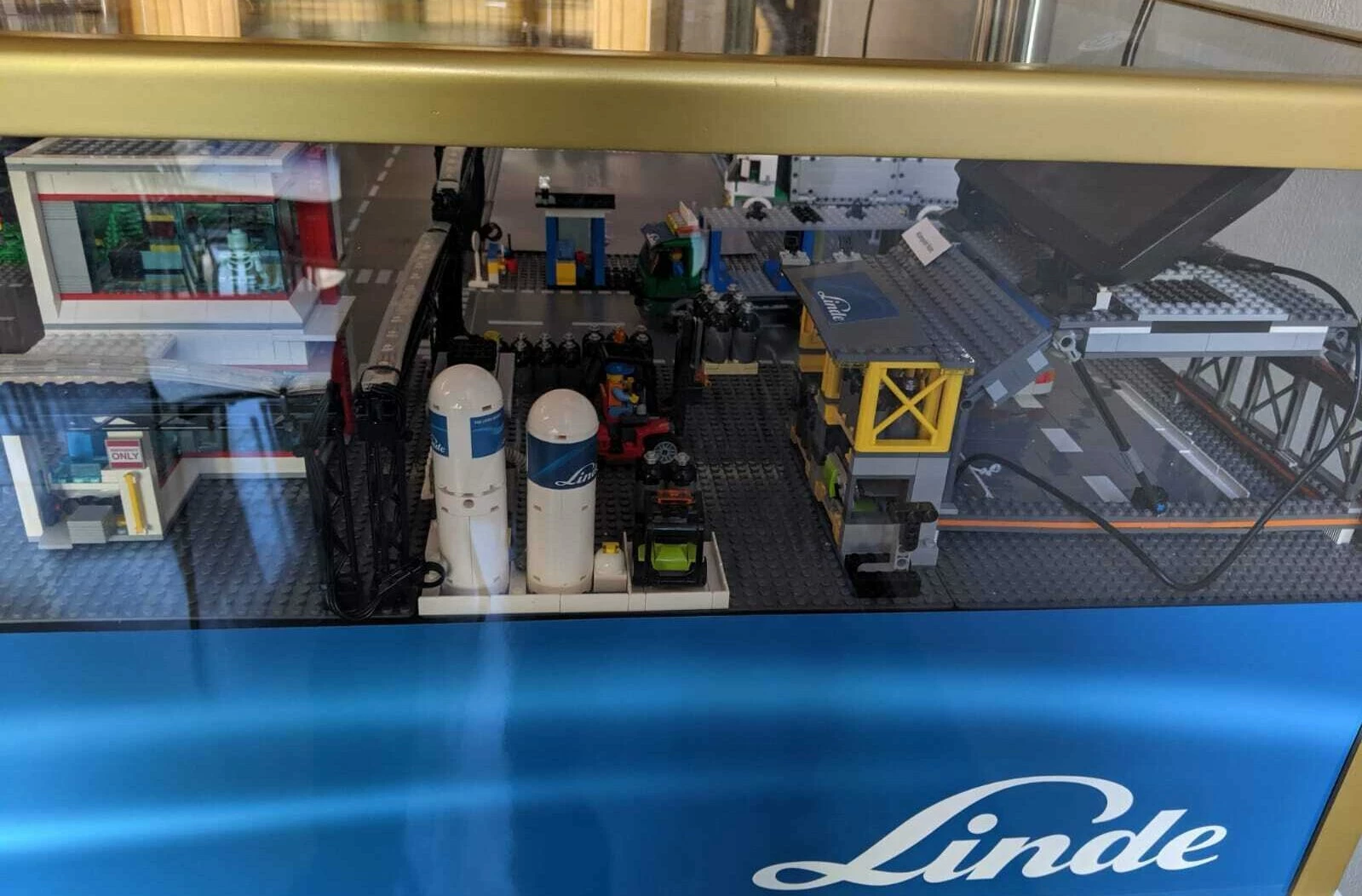
KI Wonderland for Linde
In addition, appliedAI built a miniature version of Linde's complex AI activities with Lego® to make the company's AI vision and activities more well-known among employees and the public. This model illustrates Linde's value chain and the AI activities along the processes, making Linde's digital vision tangible.
The model is publicly displayed at Linde's Munich branch. This miniature version of a Linde plant and logistics to important customers was built with a simple IoT set-up (with Raspberry Pi and Arduino) and shows some AI use cases in production at Linde. This includes, for example, a camera system, monitoring of gas cylinders, and a system that predicts the demand and required production level of the plant at any given time.
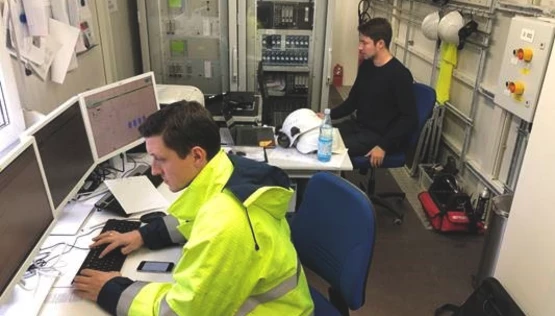
AIPlantControl
To master the journey towards AI transformation, Linde has also entered into a partnership with appliedAI. Together, the team has optimized plant operations by implementing AI into the control systems of industrial plants.
Throughout the project, Linde's enterprise-wide digital team, Linde.Digital, has spared no effort in achieving its goal. Today, it is using AI in collaboration with a team of experienced AI engineers from appliedAI. The special thing about this proof of concept is that the team created the control system directly in a real environment. It worked with an actual production plant instead of a simulator to demonstrate the potential value of the technology.
During the project, the team developed a reinforcement learning algorithm to build the AIPlantControl system. It is intended to support the operational side of the Linde business by optimizing plant operations and making them more efficient through intelligent AI controls.
To realize the AIPlantControl prototype, the team applied reinforcement learning to the existing control algorithms. Thus, it used AI to set operating parameters for valves and compressor capacity stages and achieve efficient operation through automatic intelligent controls. As a result, the system learns the behavior of Linde's process equipment and recognizes how to adjust the controllers to achieve the highest possible efficiency while reducing maintenance.
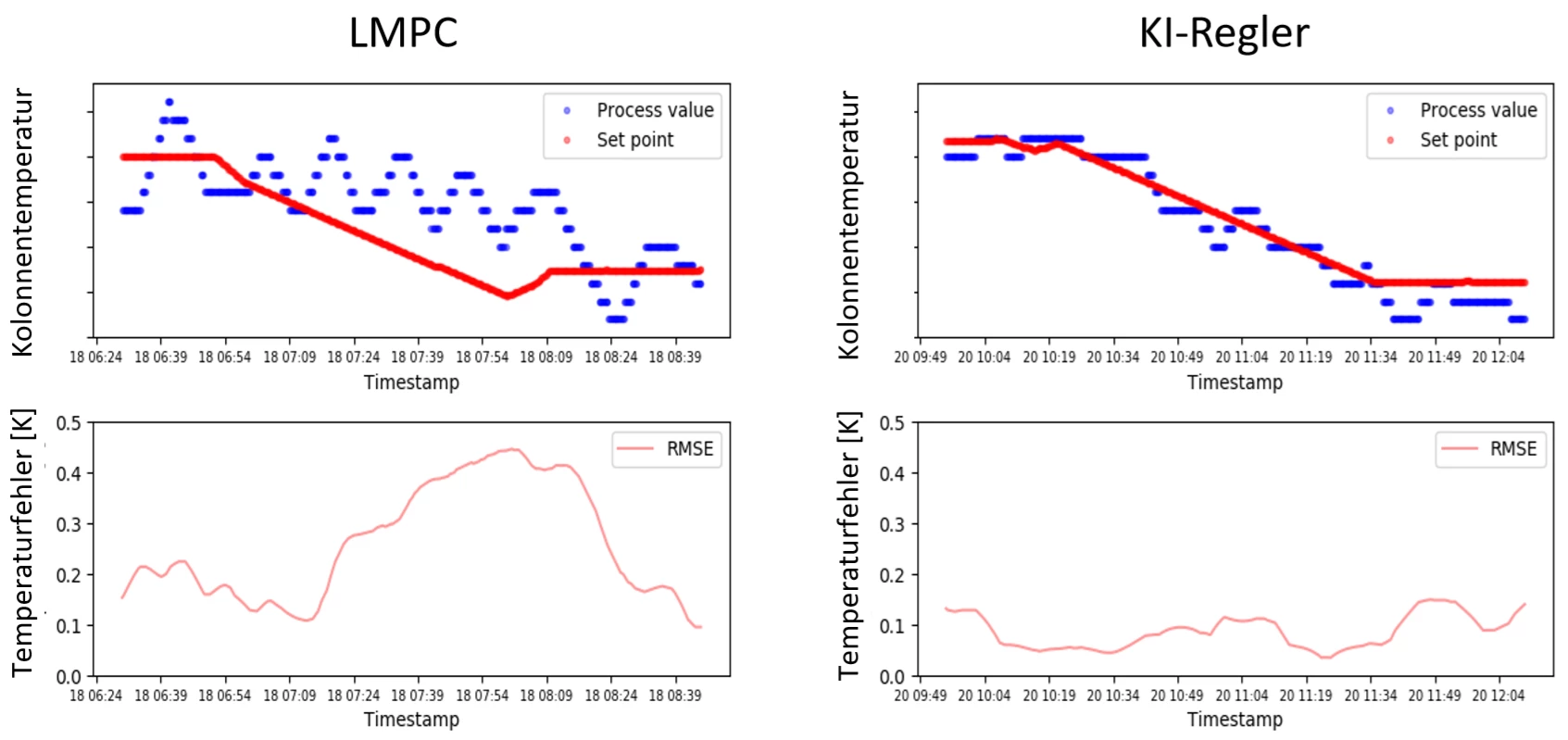
Higher Control Accuracy
Along with this measure to increase work efficiency, the team also trained the control system to imitate and adjust the physical behavior of Linde plants, achieving higher control accuracy. As the company increasingly uses renewable energy, maximum energy efficiency is possible.
This has the effect of reducing energy consumption during stable operation of the plants, as the automatic fine-tuning by the AI control system optimally adjusts the plants.
To provide a more precise example, in the AIPlantControl project, the team applied data-driven, self-learning process control to the first air separation unit (ASU) from Linde.
The attached graphic demonstrates the enormous influence that reinforcement learning can have on the control quality and efficiency of process plants. It shows how plant operation at Linde was optimized and control accuracy was increased by the AIPlantControl system. It demonstrates how the control quality differs when using optimizing AI (right side) compared to an air separation unit without AI.
Result and next steps
Looking back, we can say that the joint projects of appliedAI and Linde are already bearing fruit. The training of employees in the field of AI and the provision of AI tools has helped Linde to expand its AI team and to implement AI in its business areas.
Thanks to AI and reinforcement learning, the team developed Linde's AIPlantControl system, which optimizes plant operation.
The implemented AI-based control reduced tuning effort and improved system control accuracy. This could only be achieved through the self-learning abilities of AI. The improved control leads to optimized plant behavior and thus to savings in operating and energy costs of the plant.
For more information on all of Linde's general AI activities along the value chain, click here.
In partnership with: Linde AG
Do you have any questions about the partnership? Talk to us
Unfortunately, we cannot display the form without your consent. We use Hubspot forms that set functional cookies. Please accept functional cookies in the settings to be able to use the contact form. Or write us an email: info@appliedai.de.