In this article
- Complex Systems, Simple Solutions: The Power of Reinforcement Learning Control
- Achieving Operational Excellence: RLC Optimizes Efficiency Across Industries
- Navigating the Challenges: Integrating RLC into Existing Systems
- From Complexity to Clarity: The Human Impact of Reinforcement Learning Control
- Securing Efficiency: Achieve Remarkable Energy and Cost Savings with Reinforcement Learning Control
In the vast and intricate landscape of industrial control systems, complexity is a predominant feature. It presents numerous challenges for companies as they grapple with managing a wide network of interconnected control loops, sensors, and constraints.
The sheer multitude of variables and dependencies inherent in these systems adds to the intricacy, making optimizing system performance while maintaining operational reliability a hard task for companies. Despite concerted efforts, companies find themselves facing obstacles on their way to streamlined operations.
Complex Systems, Simple Solutions: The Power of Reinforcement Learning Control
Faced with the inefficiencies of traditional control methods, companies need a different approach to system optimization. Recognizing the urgent need for improvement, companies seek out innovative solutions that can simplify complex control processes and reduce the burden on operational staff. Reinforcement Learning Control (RLC) is the answer to these problems. It offers a powerful alternative to traditional control methods by leveraging the principles of machine learning and optimization.
Reinforcement Learning Control can transform the company's control systems by autonomously optimizing multiple control loops in real-time. Unlike traditional methods that rely on predefined rules and models, RLC adapts to changing conditions and learns from historical data to predict future behavior, enhancing both efficiency and reliability in control processes.
Achieving Operational Excellence: RLC Optimizes Efficiency Across Industries
At its core, RLC operates by iteratively adjusting control actions based on feedback from the system itself. This iterative learning process allows RLC to adapt to changing conditions and optimize performance over time. By continuously fine-tuning control strategies, RLC maximizes efficiency, reduces energy consumption, and minimizes costs.
In practical terms, this means that RLC can dynamically adjust to fluctuations in operating conditions, ensuring optimal performance across various processes. For example, in a manufacturing setting, RLC can continuously monitor and adjust production parameters based on real-time data, minimizing waste and maximizing output efficiency. Similarly, in energy distribution, Reinforcement Learning Control can analyze historical consumption patterns and environmental factors to optimize resource allocation and maintain grid stability.
Navigating the Challenges: Integrating RLC into Existing Systems
Integrating Reinforcement Learning Control solutions into existing control systems poses several challenges, primarily due to the complexity of the task. The control loops governing different systems are inherently intricate, involving numerous variables and dependencies that must be carefully considered.
One of the main challenges of implementing RLC is ensuring a smooth integration with existing control systems. This involves ensuring compatibility with various hardware and software configurations, as well as navigating the complexities of legacy systems. Additionally, the complexity of control loops requires a thorough understanding of system dynamics and interactions, further complicating the integration process.
Deploying RLC requires comprehensive training for personnel responsible for operating and maintaining the system. This means getting the staff familiar with the technical aspects of RLC algorithms and ensuring they understand how to interpret and act upon the insights generated by the system. Without proper training, there is a risk of underutilizing the capabilities of Reinforcement Learning Control or misinterpreting its recommendations, potentially leading to suboptimal outcomes.
Nevertheless, with the right training and support in place, companies can overcome these challenges and effectively utilize RLC. By investing in comprehensive training programs and providing ongoing technical assistance, organizations can empower their staff to leverage RLC effectively, optimizing performance and driving operational efficiency with RLC in their existing control systems.
From Complexity to Clarity: The Human Impact of Reinforcement Learning Control
Beyond the tangible benefits of efficiency and cost savings, Reinforcement Learning Control also has profound implications for the human element of industrial operations. By automating repetitive tasks and simplifying control strategies, RLC frees up valuable time and resources for control engineers to focus on higher-level decision-making and strategic planning. With RLC handling routine control tasks, operators can achieve greater consistency, reliability, and scalability across diverse systems and environments.
Moreover, by reducing the reliance on highly qualified staff and streamlining control processes, Reinforcement Learning Control democratizes access to advanced process control capabilities. Small and medium-sized enterprises, in particular, stand to benefit from the scalability and cost-effectiveness of RLC, leveling the playing field and driving innovation across industries.
In essence, Reinforcement Learning Control represents more than just a technological advancement; it signifies a paradigm shift in how we approach control processes in complex systems. By harnessing the power of machine learning and optimization, RLC empowers industries to achieve new levels of efficiency, sustainability, and profitability.
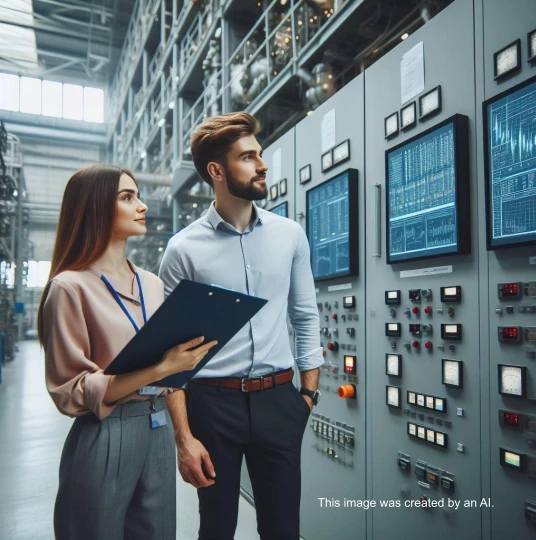
Securing Efficiency: Achieve Remarkable Energy and Cost Savings with Reinforcement Learning Control
Through the implementation of RLC, companies achieve significant benefits: efficiency gains and decreased dependence on highly qualified staff. However, another compelling benefit of RLC is its ability to drive significant energy and cost savings, while also improving system reliability and performance. In industries where energy consumption accounts for a substantial portion of operational expenses, even minor improvements in efficiency can yield substantial financial benefits.
Use cases for Reinforcement Learning Control include controlling high temperature processes like steel and glass production and dryers, managing cryogenic processes such as air separation and liquefaction, optimizing building control including data centers for energy management, and overseeing complicated processes with many unknown disturbance sources, such as polymer production, tunnel boring, and pipeline control.
Implementing RLC in these areas can lead to significant efficiency gains, with potential reductions in energy consumption ranging from 30% to 55% within the first year, depending on the use case and the level of control optimization already achieved.
Energy reduction in different industries
Consider the case of a non-optimized production plant, where traditional control methods struggle to cope with the complexities of the system. Through precise control and optimization of heating processes, RLC checks that energy is used more efficiently, minimizing waste and maximizing productivity. As a result operators can achieve energy savings between 40% to 60%.
Similarly, in sectors like chemical manufacturing, where process optimization is critical, RLC can push systems closer to their physical limits while maintaining output quality. As a result, operators in this sector can achieve energy savings ranging from 5% to 10%.
Even in industries like data centers, where cooling systems play a crucial role in maintaining optimal operating conditions, RLC offers significant advantages compared with traditional control methods. Operators, then, can reduce energy consumption by 20% to 40%.
Download a comprehensive summary and share it within your team
Unfortunately, we cannot display the form without your consent. We use Hubspot forms that set functional cookies. Please accept functional cookies in the settings to be able to use the contact form. Or write us an email: info@appliedai.de.