Looking at the application of artificial intelligence and machine learning, a big chasm seems to be opening up: On the one side there are most of the tech companies where applying machine learning is the norm. In fact, the business models of companies such as uber, Airbnb, Zalando or even TikTok would no longer function today without the use of machine learning. On the other side there are large companies that, amidst the Covid-19 pandemic, continue to heavily invest into data and AI initiatives (NewVantage Partners), but only a minority report significant business impact of their initiatives. A large number of initiated ML projects remain stuck at a PoC level and fail to reach the hurdle of going into production - studies report failure rates between 80% and 90%.
What is the reason for this lack of impact in “traditional” enterprises and the high share of failed projects? Certainly, there is a variety of reasons, e.g. the competition for skills and talents, the adoption of new technologies, and, of course, it's easier to apply machine learning when you have a fully digital product and no legacy IT systems. But above all, one major reason that we observe with increasing frequency is the lack of an AI product mindset. Many AI initiatives forget to consider what value an initiative creates for the users as AI has inherent characteristics that make accurate planning quite difficult in the early stage of the initiative.
Challenges as such address two types of people in today’s teams:
Firstly, AI people are currently mostly equipped with technical skills and typically lack knowledge from the domain of digital product management with its processes and methods.
Secondly, product managers need to understand the unique requirements of data products and how machine learning features are developed as they will to some extent be part of any digital product in the future.
We argue that there is a need for a dedicated discipline and role to make data-driven products successful. We call this role the data product manager.
A user cares whether their problem is solved - not if a machine learning model has 90% or 95% accuracy
AI initiatives in “traditional” enterprises are often organized as projects - even if the goal is to develop a “data product”. They largely follow a structure similar to other initiatives in large enterprises: A predefined scope should be delivered against specific budget constraints and time limitations. The initiative is considered successful the moment the desired output is generated. Equally, machine learning projects are often considered successful if a model can predict something with a certain accuracy.
What is wrong with this and why? Coming from the world of data product management, projects can decoy you into a trap: Many data initiatives and developments - managed in a project mode - bear risks to forget the user. And the moment this happens, it finally leads to the situation where organizations struggle with truly adopting data products into their business processes, products or services. So, the finalized projects just peter out without creating any business outcomes, without adding real value to their users or businesses, and without fueling the organization’s data culture.
Certainly, the realization of putting the user first is neither new nor specific to AI. However, there are inherent properties of AI that make the use of a product mindset an absolute must:
Firstly, in the world of AI and ML, organizations can only judge the full potential outcome of a product or solution after the development phase. Therefore, they can only partially define typical project parameters before the start of the development phase, such as a-priori specified outputs, a predetermined end date and a fixed budget. Therefore, having a product mindset, the planning process reflects rather the conception of a product, using product discovery methods and assumptions.
Secondly, AI solutions are never “done” or “finally delivered”. They require continuous delivery and operations comparable to a product lifecycle. As such, organizations need to put a dedicated team (or teams) in place that is responsible for a specific AI product - just like they do it for regular products. The team is responsible for the product’s ongoing development and its complete integration and adoption within the organization.
Thirdly, in order to fully exploit the value from data products, they must be integrated into a greater ecosystem, e.g. a digital product or a platform. Feature integration into overall ecosystems is a typical task in digital product management.
To sum up, the development of AI and machine learning solutions should be considered as a “real product” in order to be successful. And “products” does not only include services that are offered to external customers, but also internal products or services - just any type of initiative that is supposed to create value from data.
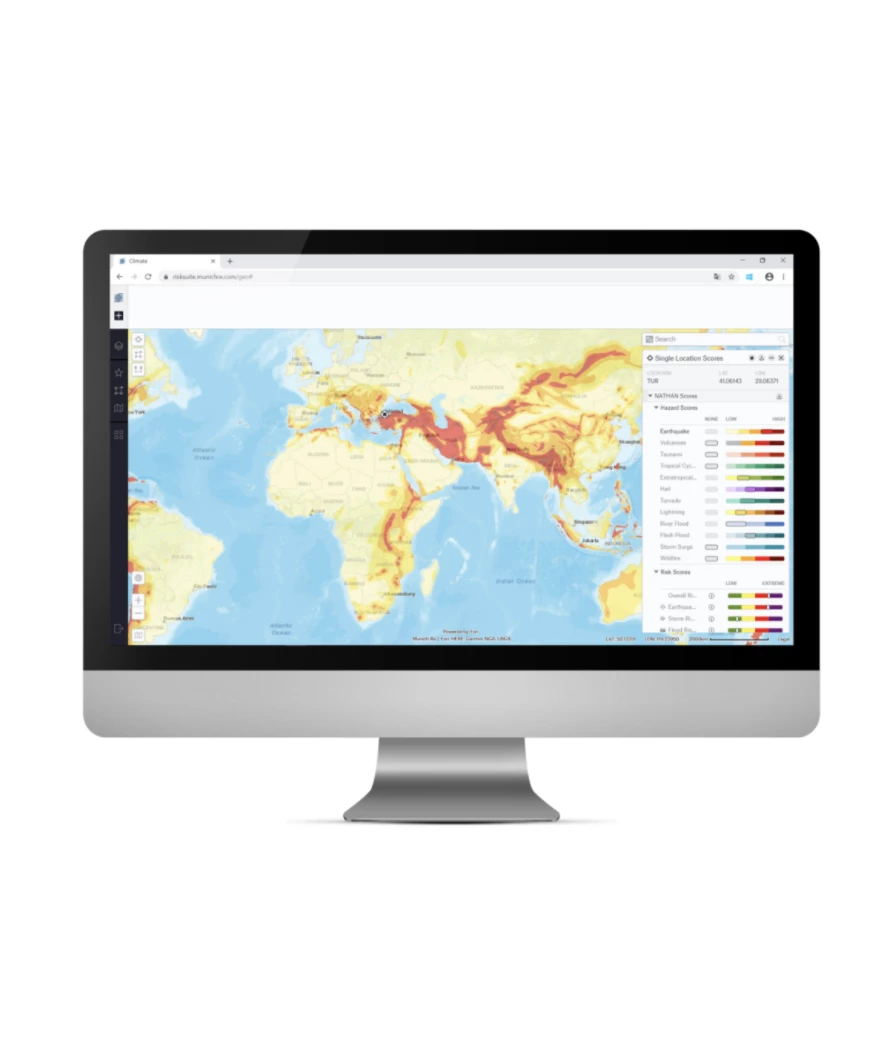
Leveraging the product mindset for Munich Re’s Location Risk Intelligence Platform
The benefit of adopting a product mindset is also clearly demonstrated by examples from the leading reinsurance company in the world Munich Re.
With the goal to provide a completely new customer experience, Risk Management Partners a division, specialized in risk management solutions and services, of Munich Re launched its unique SaaS solution for risk assessment and management capabilities for addressing physical and financial risks to which companies are exposed as a result of natural catastrophes and climate change. After a successful Go-to-market in 2019, the need for data product lifecycle management rapidly came into focus. By implementing a custom-fitting data product management approach, Risk Management Partners has been put into the position of continuous product operation while further developing the solution in a modern way. The entire product team learned to hold up competitive advantage and how to address customer needs at the same time to keep satisfaction high.
By establishing cross-functional teams, data product managers, product owners, data scientists and software engineers acting as one team, increasing the customer base significantly within the last 12 months and driving business for both their customers and Munich Re in a successful collaboration. Turning from a project organization to a product organization and implementing data product management facilitated Risk Management Partners to keep track of continuously discovering new features and delivering customer outcomes in a business model truly based on data.
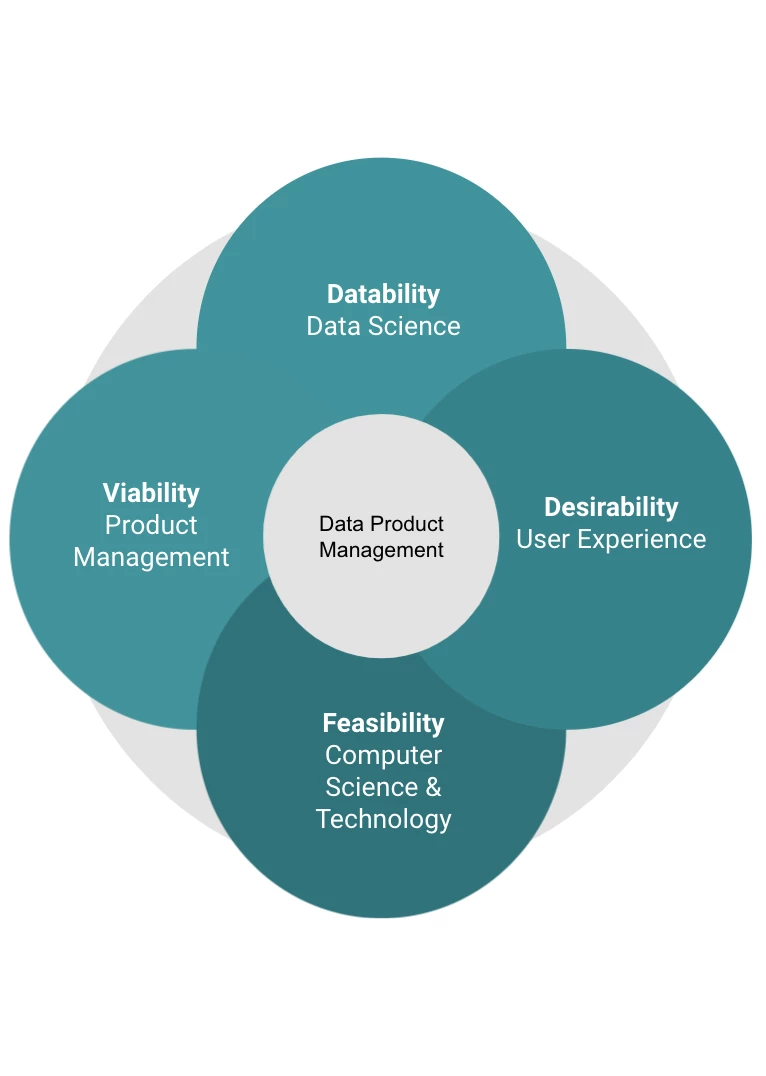
Responsible for the value creation process - the role of a data product manager
Whenever working with data science teams, it quickly turns out: the discipline of AI and ML is part of the engineering science profession. Data scientists and ML engineers are used to focusing on the technical feasibility of the solutions they develop. This perspective sometimes blocks the view for other factors that ensure success, e.g. whether the business case is compelling or what it is that users or customers actually need.
Thinking about solutions from a business point of view and from a user perspective is what product management is all about. The mantra of product management always has been the balance of the three dimensions named as feasibility, viability and desirability. While each dimension requires different skills and contains different tools and methods, its combination often results in product success and thus generates business outcomes.
Applying the product management discipline to data products, it becomes evident that the ecosystem demands an additional dimension for product managers: datability. This dimension addresses the need for a profound knowledge and skill set in the field of data. The perspective of datability assesses data potentials of an existing product idea and integrates the identified assumptions into the product management mantra explained above. So, by the combination of feasibility, viability, desirability and datability, a data product can be conceptualized, designed, and realized in a proper and successful manner.
Setting up the right team to tackle AI products with a product mindset
So, let’s build a future product crew - a cross-functional team. To be able to create value for users and customers, we first need to specify the environment in which the AI model should be integrated: will it be integrated into another system like a digital product or a platform? Will it be deployed to a certain endpoint to be consumed, e.g. in the form of a dashboard or a technical service? Will it be embedded as a micro service into a respective architecture?
Let’s assume we want to integrate it into a digital product, which requires a strong collaboration with the responsible product team. It leads to the following team set-up:
- Data product manager: builds the bridge into the digital product team by aligning the business case assumptions within the related business model, understanding customers’ needs and desires, explaining the potential data capabilities while working closely with the data science team.
- Data product owner: the data product owner is responsible for the realization of the data product together with the data science team. They own the development and ensure that the product meets all requirements that come from the business space. They are in close collaboration with the data product manager and aligns with them intensively.
- UX designer: a UX designer supports the responsible team of data product manager and data product owner to understand the customer’s needs in a structured way. They create customer insights, tests and validates them, and translates them into clear technical requirements and implementation concepts. At this point, it is important to understand that, in order to meet the user’s expectations towards the later product, it is not only about the visual design of the user interface (the frontend), but about the entire user experience.
- Data Scientist: Data scientists juggle with data. More specifically, they derive insights from the vast amount of data available within the organization. Using their excellent statistical understanding, they conduct analyses and discover patterns and structure in data. They can validate and present data-driven use cases and hypotheses.
- ML-engineer: ML engineers implement the AI systems. They have technical responsibility for the overall solution and manage the scalability, reproducibility, and overall quality of an AI product.
- Data Engineer: the data engineer works closely with the data scientist and ML-engineer taking responsibility for so-called data pipelines, which extract data from its sources and store it in the adequate concept. In most cases, they also own the data architecture and ensure the technical realizability of AI on the available technical stack.
- DevOps Engineer: the DevOps engineer builds the technical bridge towards the digital product team. In close cooperation with its team members, they take responsibility for the integration part, tests the data product end2end, and aligns with the technical team from the digital product.
How can you trigger the process of creating an AI product with a product mindset?
Turning from output to outcome and from project to product is a huge step. It involves topics like change, high degree of structure, and sense for process operations. It’s a mindset shift and therefore requires discipline and consistency among all stakeholders. If you just feel the need to start with a product mindset for your AI products, we suggest three simple things to start with:
- Name and specify the outcome you want to generate: the discussion about outcomes is the first right step. Brainstorm and discuss what actual outcome you want to see at the end of your process and who will benefit from it. Define an objective or a KPI to make it tangible.
- Assess your team set-up with focus on activities: Each product is different, and each use case requires different skills. Therefore, teams always have slightly different roles as defined in theory. While roles may be different, the required activities to deliver the product are not and thus teams need to be designed based on the number of activities to cover. While in smaller products, activities are covered by one role, e.g. the data scientist covers activities of the ML-engineer or the data product owner covers activities of the data product manager, roles need to be split whenever the product scales.
- Design and introduce data product lifecycle process: the real work starts whenever the data product has been launched and users or customers consume it. They will provide feedback (if you are lucky), which needs to be integrated back into your development process. The degree of professionalism in your lifecycle management and the continuity you can build up will heavily influence the outcome creation process and thus its perception for the recipient.
The moment you achieve the operationalization of outcome creation by various methods and tools existing, a product mindset will sooner or later arise and establish - and data product success will grow. Above all, your user or customer will thank you for it.