AI-Driven Efficiency: Revolutionize your Information Management with RAG
Fragmented information scattered across multiple systems and repositories slows down productivity and makes collaboration harder. RAG is the solution that applies natural language queries to access information from internal company data sources, and ultimately simplifies the workflows.
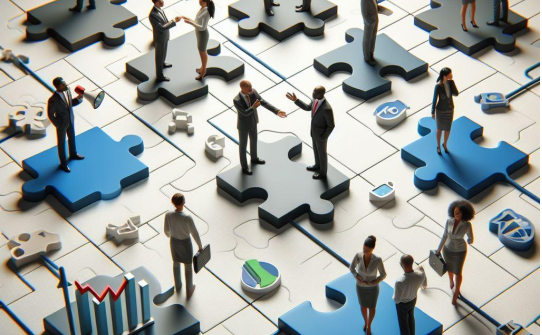
In this article
- The Challenge of Information Overload
- Navigating Complexity: The Struggle for Efficiency
- Empowering Customers: AI Insights Drive Integration and Data Privacy
- How Retrieval Augmented Generation Works
- Empowering Employees: The Promise of Workflow Automation
- Realizing Efficiency Gains: The Economic Impact of RAG Implementation
In many organizations, information is scattered across multiple systems and repositories. Employees may spend valuable time searching for relevant data, only to be met with disparate sources and formats. This fragmentation not only hampers productivity but also inhibits collaboration and innovation.
For companies operating in highly regulated industries or complex environments, the challenge is even greater. Traditional methods of information retrieval and analysis fall short in the face of dynamic data landscapes and evolving business requirements. As the volume and complexity of data continue to grow, organizations must find new ways to extract actionable insights and drive value.
The Challenge of Information Overload
Traditional solutions for managing and accessing data often rely on structured databases or document management systems. While these tools may provide some level of organization, they lack the flexibility and adaptability required to meet the diverse needs of modern businesses. Moreover, the manual effort required to maintain these systems can be resource-intensive and prone to errors.
Navigating Complexity: The Struggle for Efficiency
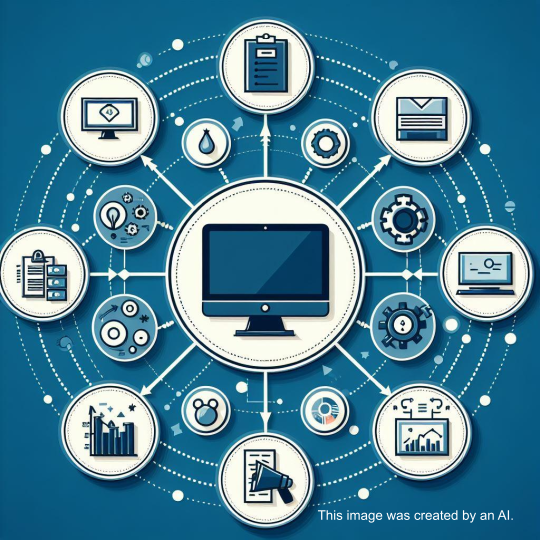
In their pursuit of clarity, companies come across Large Language Models that offer relevant use case portfolio management and strategy support. They need LLMs solutions (such as ChatGPT) but customized specifically to support them dealing with their own internal data so that the users can make well-informed decisions. In this respect, LLMs act as advisors.
The AI interface is designed to be sleek and straightforward, resembling a web application, which facilitates easy interaction for users. The solution applies natural language queries to access information from internal company data sources. When no data is available, the solution keeps transparency notifying the absence of information on the topic rather than providing speculative or false answers.
Empowering Customers: AI Insights Drive Integration and Data Privacy
As soon as the Large Language Model (LLM) is customized to fit the company's needs, it must be integrated into existing infrastructure and workflows. Yet, this integration presents its own challenges: the team has to make sure of technical compatibility while integrating the custom LLM with various software applications, databases, and communication platforms used across different departments.
In addition, responsible team members have to pay attention in transferring relevant data to the new solution to prevent data format discrepancies or loss. Therefore, user training and adoption are equally critical, as employees need to be proficient in using the LLM. As resistance to change can hinder progress, change management is essential to mitigate disruptions to existing processes and workflows.
Another crucial topic is data security as well as compliance. Data privacy should be prioritized with customizable settings to safeguard sensitive information. That’s why - through several clearance levels - stringent required measures must be set in place to protect sensitive data that the LLM processes.
Collaborative efforts in monitoring and optimizing the LLM’s performance between IT teams, data scientists, end-users, and management stakeholders are necessary not only for a successful integration but also to plan for scalability and long term viability.
How Retrieval Augmented Generation Works
At its core, RAG combines the power of information retrieval with the creativity of language generation. What sets RAG apart from traditional solutions such as search engines, database queries, and unstructured documents, is its ability to handle complex queries and generate contextually relevant responses. Specifically, rather than simply retrieving documents or data points, RAG understands and interprets the intent behind the query formulated in natural language, and generates human-like responses that are tailored to the user's needs.
Empowering Employees: The Promise of Workflow Automation
While the technical capabilities of RAG are impressive, its true value lies in its ability to empower employees and enhance collaboration within organizations. Through a streamlined process of accessing and sharing information, RAG helps teams work more efficiently and effectively.
With RAG, employees no longer need to spend hours searching through documents or databases to find the information they need. Instead, they can simply ask a question in natural language and receive a timely and accurate response. This not only saves time but also reduces frustration and improves morale.
RAG and Data Privacy: Balancing Security and Accessibility
Of course, with great power comes great responsibility, and organizations must watch over the protection of sensitive data. In this respect, RAG offers customizable privacy settings that allow each company to control access to sensitive data based on user roles and permissions.
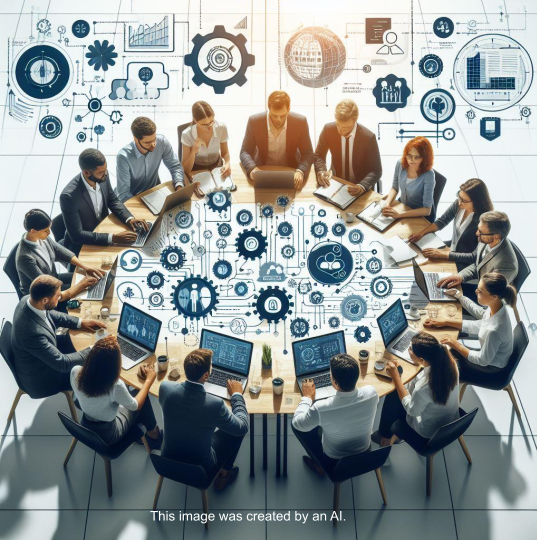
Realizing Efficiency Gains: The Economic Impact of RAG Implementation
Studies have shown that organizations that adopt RAG can achieve significant improvements in productivity, with some companies reporting increases of up to 45% in function costs.
Beyond productivity gains, RAG also has a substantial economic impact, reducing costs and increasing revenue for organizations that leverage its capabilities.
As organizations continue to evolve and adapt to changing market conditions, the role of RAG in driving innovation and growth will only continue to grow. By investing in RAG and embracing its transformative potential, companies can position themselves for long-term success and leadership in their respective industries.